StreamingContext: Spark streaming word count example Scala
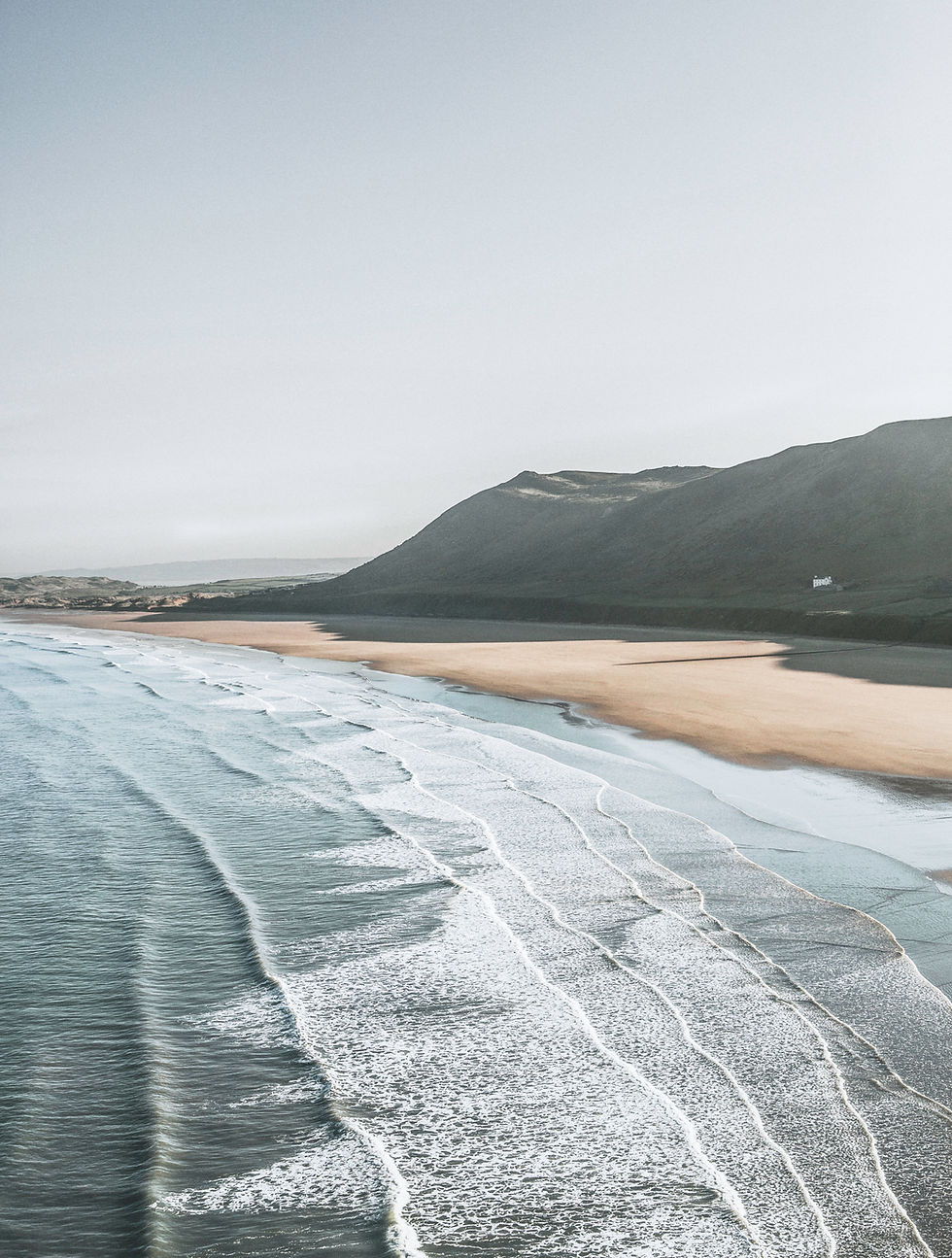
Main menu: Spark Scala Tutorial
In this tutorial you will learn,
How to stream data in real time using Spark streaming?
Spark streaming is basically used for near real-time data processing. Why I said "near" real-time? Because data processing takes some time, few milliseconds. This lag can be reduced but obviously it can't be reduced to zero. This lag is so minute that we end up calling it real-time processing.
Streaming word seems very cool but honestly speaking most of you have already implemented this in the form of "batch mode". Only difference is the time window. Everyone is aware of batch mode where you pull the data on hourly, daily, weekly or monthly basis and process it to fulfill your business requirements.
What if you start pulling data every second and simultaneously you made your code so efficient that it can process the data in milliseconds. It would be automatically near real time processing, right?
Spark streaming basically provides you ability to define sliding time windows where you can define the batch interval.
After that, Spark automatically breaks the data into smaller batches for real-time processing.
It basically uses RDD's (resilient distributed datasets) to perform the computation on unstructured dataset.
RDD's are nothing but references to the actual data which are distributed across multiple nodes with some replication factor which reveal their values only when you perform an action (like collect) on top of it, called lazy evaluation.
Screen 1
Open Scala IDE, create package com.dataneb.spark and define Scala object SparkStreaming. Check this link how you can create packages and objects using Scala IDE.
Copy paste the below code on your Scala IDE and let the program run.
package com.dataneb.spark
/** Libraries needed for streaming the data. */
import org.apache.spark._
import org.apache.spark.streaming._
import org.apache.spark.streaming.StreamingContext._
object SparkStreaming {
/** Reducing the logging level to print just ERROR. */
def setupLogging() = {
import org.apache.log4j.{Level, Logger}
val rootLogger = Logger.getRootLogger()
rootLogger.setLevel(Level.ERROR)
}
def main(args: Array[String]) {
/** Defining Spark configuration to utilize all the resources and
* setting application name as TerminalWordCount*/
val conf = new SparkConf().setMaster("local[*]").setAppName("TerminalWordCount")
/** Calling logging function */
setupLogging()
/** Defining spark streaming context with above configuration and batch interval as 1*/
val ssc = new StreamingContext(conf, Seconds(1))
/** Terminal 9999 where we will entering real time messages */
val lines = ssc.socketTextStream("localhost", 9999)
/** Flat map to split the words with spaces and reduce by key pair to perform count */
val words = lines.flatMap(_.split(" "))
val pairs = words.map(word => (word, 1))
val wordCounts = pairs.reduceByKey(_ + _)
// Print the first ten elements of each RDD
wordCounts.print()
ssc.start() // Start the computation
ssc.awaitTermination() // Wait for the computation to terminate
}
}
Screen 2
Now open your local terminal and type "nc -lk 9999" , hit enter. What we did just now? We just made a network connection (nc or NetCat) to local port 9999.
Now type some random inputs for Spark processing as shown below,

Screen 1
Now go back to Scala IDE to see the processed records, you need to swap the screens quickly to see the results as Spark will process these lines within seconds.
Just kidding, you can simply pin the console and scroll back to see the results. You can see the output below.
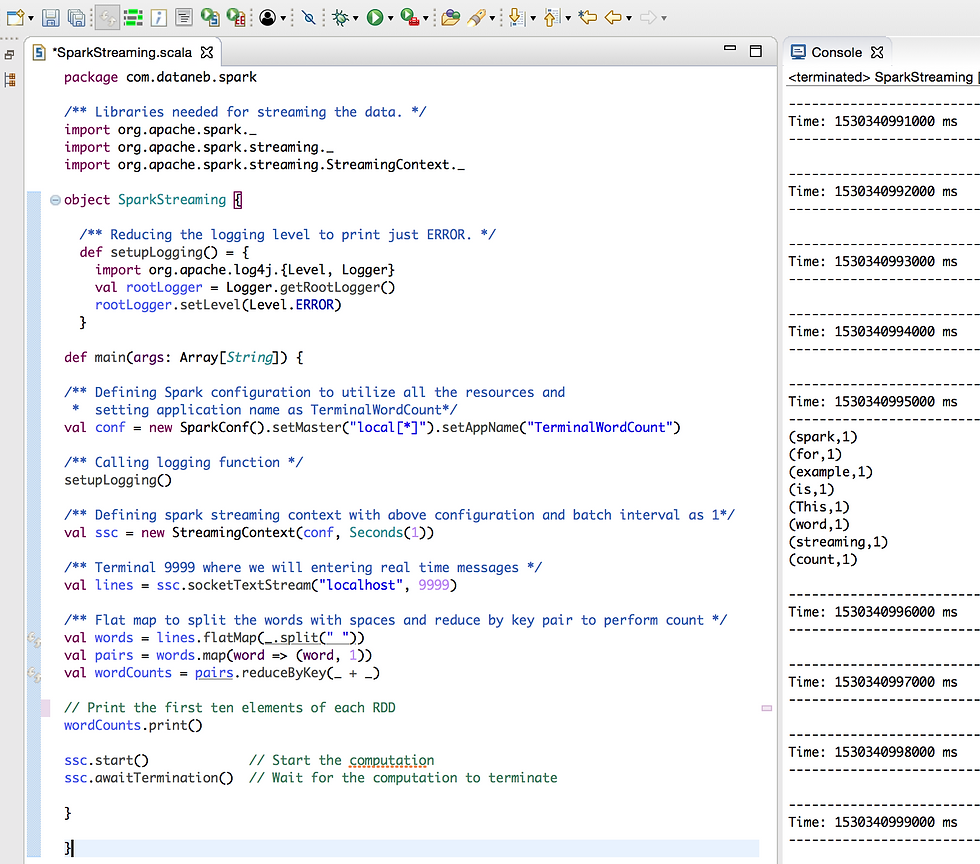
That's it. It's so simple. In real life scenario you can stream the Kafka producer to local terminal from where Spark can pick up for processing. Or you can also configure Spark to communicate with your application directly.
Thank you!! If you have any question write in comments section below.
Navigation menu
1. Apache Spark and Scala Installation
2. Getting Familiar with Scala IDE
3. Spark data structure basics
4. Spark Shell
5. Reading data files in Spark
6. Writing data files in Spark
7. Spark streaming
9. What's Artificial Intelligence, Machine Learning, Deep Learning, Predictive Analytics, Data Science?
10. Spark Interview Questions and Answers
Comments